Big Data’s Radical Potential
Today, big data is used to boost profits and spy on civilians. But what if it was harnessed for the social good?
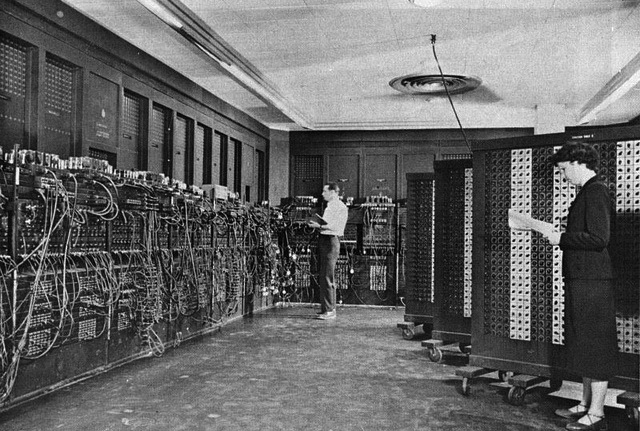
ENIAC, the first general-purpose computer. US Army
The software giant Oracle has big claims about “big data.” They call it “the electricity of the twenty-first century — a new kind of power that transforms everything it touches in business, government, and private life.”
There is no consensus about what “big data” is, exactly, but fans and critics agree that it’s reshaping the way we live. Advocates confidently predict that ever-increasing volumes of complex data — combined with new techniques for storage, access, and analysis — will revolutionize everything we do, from basic scientific research to the way social interactions are organized. Prominent data scientists such as Alex Petland say that we are witnessing a fundamental social transition to a new “data-driven” society that has the potential to be “more fair, stable, and efficient.”
The skeptics are equally emphatic. The anti-consumerist magazine Adbusters recently pronounced the death of nation states, “stripped by the global machine of finance, computation, and all pervading Big Data algorithms.” Pam Dixon, executive director of the World Privacy Forum and co-author of a chilling new report on secret consumer scores, argues that we may be on the verge of the dystopian future described by Philip K. Dick in stories like The Minority Report where predictions from big data algorithms can “become someone’s destiny.”
So what is big data — and who are we to believe?
No one thing distinguishes big data from old-fashioned, “small” data. Mass-scale data is not new — even in 1924, the Eugenics Record Office at Cold Spring Harbor had over 750,000 records tracing the “inborn physical, mental, and temperamental properties” of American families. The Social Security Administration was already tracking 26 million people, using over 500,000 punch cards a day in the 1930s.
What makes big data different from these projects is the scale and scope of what’s being gathered and analyzed, often summarized by the “3 Vs”: volume, variety, and velocity. The volume of data being generated today is astounding. There are over 3 million data centers worldwide, with US data centers using an estimated 2 percent of all power nationwide. Americans upload more than two hundred hours of video every minute and over 500 millions photos each day, and digital information from emails, banks transactions, the worldwide web, medical records, and smartphone apps increases every day.
Quantity is matched by faster, more efficient processing. In 2012, Facebook processed over 500 terabytes of data a day — fifty times the entire printed collection of the US Library of Congress. These trends are expected to continue well into the future. As more devices are embedded with wired and wireless sensors and can independently communicate with each other and centralized data repositories without human intervention in the so-called “internet of things,” the volume and variety of data sources will increase dramatically.
The appeal of big data is straightforward. For governments, the potential to rationalize policies and actions, whether that’s identifying perceived political threats or changing educational outcomes in classrooms, is alluring. For corporations, the statistical relationships uncovered by big data offer new avenues for profit making. Data-driven practices, its corporate advocates say, can increase productivity, cut costs, accurately target potential customers, and even uncover new markets.
But what the big data hype misses is that collecting large amounts of raw data is not in itself useful or profitable. It’s the promise of what you can do with the data — and the statistical relationships that can be gleaned from its analysis — that really matters.
These relationships can be scientific (the optimal dosage of the anti-coagulant drug Warafin is correlated with particular genetic variants of an enzyme that processes Vitamin K); or political (people who are members of an Evangelical church are much more likely to vote Republican); or commercial (shoppers who buy large quantities of specific products are likely to be pregnant).
In fact, the New York Times recently explained how Target used these kind of statistical relationships to develop a pregnancy score that predicts how likely a shopper is to be pregnant. The models can be shockingly quantitative:
Take a fictional Target shopper named Jenny Ward, who is 23, lives in Atlanta and in March bought cocoa-butter lotion, a purse large enough to double as a diaper bag, zinc and magnesium supplements and a bright blue rug. There’s, say, an 87 percent chance that she’s pregnant and that her delivery date is sometime in late August.
But extracting such meaningful statistical relationships from large, unstructured datasets is not an easy task.
Numerous thorny problems arise: how can we identify the important features that define some dataset, even when we do not know these features ahead of time (unsupervised learning)? How can we organize and visualize the information contained in a large dataset (data visualization)? How can we learn statistical relationships between different features in the data (supervised learning)? How can we devise statistical methods to recognize predetermined patterns in the data (pattern recognition)? These are the central concerns of the emerging disciplines of machine learning and data science, as well as modern statistics.
One of the principal lessons from recent research in statistics and machine learning is that there is no such thing as a perfect big data algorithm — every statistical procedure makes errors. Error is unavoidable because there is a fundamental trade-off in any statistical procedure between generalizability — the ability to make accurate predictions — and the ability to optimally explain existing datasets. The larger and more complex the data being analyzed, the more difficult it is to navigate these tradeoffs.
To illustrate this point, consider a hypothetical statistical procedure that has the goal of predicting whether a YouTube video contains a cat. The input to the procedure is a video clip, and the output is a binary prediction: a “yes” if the procedure predicts the video contains a cat and a “no” if it predicts it does not.
The procedure works by “learning” a statistical model that can distinguish between videos that do and do not contain cats. To learn the model, the procedure is trained on training data (for example, a large collection of labeled YouTube videos with and without cats). The goal is to create a model that can make predictions about new videos that are not in the training data.
During the training process, the parameters of the statistical procedure are optimized to maximize the predictive performance of the procedure. Since eliminating error is impossible (due to inherent tradeoffs involved in analyzing big data), the person training a statistical procedure must make a choice about the types of errors they will allow.
They can choose to minimize the number of false positives (videos without cats incorrectly classified as having cats), or the number of false negatives (videos with cats incorrectly classified as not having cats), or the total number of misclassified videos (videos without cats incorrectly classified as having cats as well as videos with cats classified as not having cats).
The details of how someone trains a statistical procedure to find cats seem rather abstract and mundane. Yet, when governments and corporations use big data to turn social relationships into mathematical relationships, these mundane statistical details can have horrific consequences.
If instead of training statistical procedures to find cats on YouTube the goal is to train an algorithm to identify “militants” in surveillance videos for targeted assassination (i.e. signature strikes), a higher false positive rate means killing innocent people, not missing cats.
These unavoidable tradeoffs ensure that in any statistical algorithm, minimizing the number of false negatives (misclassifying “terrorists” as civilians) will increase the false positives (civilians who are mistakenly assassinated).
So when the government recently loosened its stated policy from requiring that the military “ensure” that civilians not be targeted to requiring the military “avoid targeting” civilians, the outcome is inevitable: more false positives and more innocent people killed.
Thus, despite the military’s repeated claims to the contrary, it is not surprising when drones mistake wedding processions for militant convoys and turn weddings into funerals. This is the unavoidable collateral damage of treating social relations as abstract statistical objects.
The antisocial consequences of fetishizing big data are not confined to the military. Big data is now a valuable commodity, used by corporations to predict people’s spending behaviors, health status, profitability, and much more.
These statistical algorithms have given rise to a plethora of secret consumer scores: the “Consumer Profitability Score,” an “Individual Health Risk Score,” “Summarized Credit Statistics” that score people based on their zip code for financial risk, “fraud scores,” and many more.
These statistical scores often mask complex social relations. As the World Privacy Forum succinctly summarized: “Secret scores can hide discrimination, unfairness, and bias.” More fundamentally, the scores turn data about our personal lives into a commodity, the end goal of which is corporate profitability.
A striking example of using big data in pursuit of corporate profits is the adoption of big data algorithms to schedule workers. Scheduling software, based on sophisticated statistical models that incorporate a myriad of factors like historical sales trends, customer preferences, and real-time weather forecasts, enable companies to schedule workers down to the minute. Shifts are scheduled in fifteen-minute blocks and change daily, ensuring there are just enough workers to meet the anticipated demand. Corporations are reducing hours while simultaneously increasing the intensity of work performed by its employees.
The inevitable consequence is that workers’ lives become dominated by the statistical logic of profit maximization. Workers have no fixed schedules and no guarantee of hours. As a recently profiled Starbuck’s worker explained, statistical algorithms dictate everything in her life, from how much sleep her son gets to what groceries she buys in a given month.
Yet, the same data and algorithms that wreak havoc on workers’ lives could just as easily be repurposed to improve them. Worker cooperatives or strong, radical unions could use the same algorithms to maximize workers’ well being.
They could use the data on sales trends, preferences, and weather to staff generously during peak hours so that workers get adequate breaks and work at a more reasonable pace. It’s simply a matter of changing what is optimized by the statistical procedure: instead of optimizing a mathematical function that measures corporate profitability, the function could be changed to reflect workers’ welfare.
The emancipatory potential of big data is easiest to see in the context of basic biological research. Over the last fifteen years, big data techniques based on high-throughput DNA sequencing have transformed biological research, allowing scientists to make progress on numerous fundamental problems that range from how chromosomes are arranged inside cells, to the molecular signature of cancer, to identifying and quantifying the billions of bacteria that live in and on your body.
As these examples show, when the profit motive is muted, big data can be readily harnessed for the benefit of society as a whole. (Though it is worth pointing out that Big Pharma and other corporate interests are methodically trying to privatize any potential benefits from the bioinformation revolution. Hilary Rose and Steven Rose discuss this at length in their new book, Genes, Cells, and Brains.)
Big data, like all technology, is imbued within social relations. Despite the rhetoric of its boosters and detractors, there is nothing inherently progressive or draconian about big data. Like all technology, its uses reflect the values of the society we live in.
Under our present system, the military and government use big data to suppress populations and spy on civilians. Corporations use it to boost profits, increase productivity, and extend the process of commodification ever deeper into our lives. But data and statistical algorithms don’t produce these outcomes — capitalism does. To realize the potentially amazing benefits of big data, we must fight against the undemocratic forces that seek to turn it into a tool of commodification and oppression.
Big data is here to stay. The question, as always under capitalism, is who will control it and who will reap the benefits.