Israel’s War Crimes in Gaza Show the Real Danger of AI
The most pressing menace from artificial intelligence is not a sci-fi scenario where machines take over from human beings. It’s the use of AI as a tool to carry out atrocities that are 100% man-made — something we can see playing out right now in Gaza.
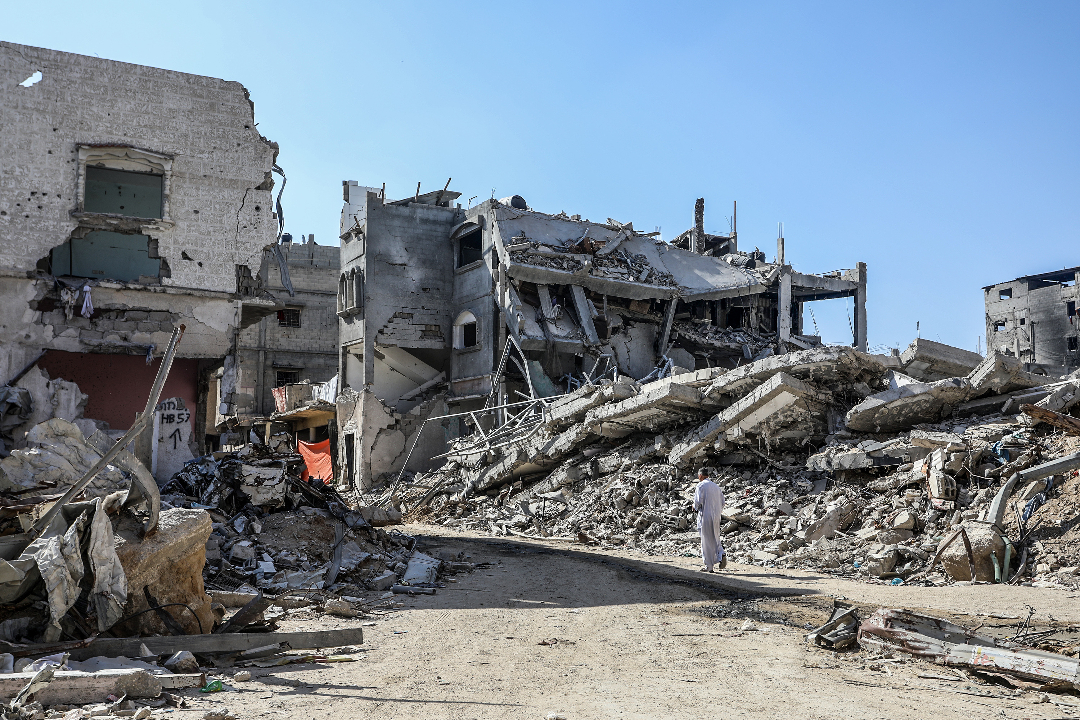
A view of the destruction after the withdrawal of Israeli forces from Khan Yunis, Gaza, as some Palestinian residents began to return to their homes on July 18, 2024. (Abed Rahim Khatib / Anadolu via Getty Images)
For a brief moment in 2023, the biggest existential threat to humankind seemed to come not from anthropogenic climate change but another human-driven specter: artificial intelligence. Ushering in this new dystopian flavor was OpenAI’s launch of ChatGPT-3, a large language model, or LLM, capable of generating long sequences of predictive text based on human prompts.
In the following weeks, as people across the world consumed billions of watts of energy submitting prompts like “Rewrite the Star Wars prequels where Jar Jar Binks actually is the secret Sith Lord,” the public discourse became congested with a slurry of technological prognostications, philosophical thought experiments, and amateurish science-fiction plots about sentient machines.
Major media outlets published op-eds like “Can We Stop Runaway A. I.?” and “What Have Humans Just Unleashed?” Governments across the West scrambled to form oversight committees, and every tech-savvy person adopted a new vernacular almost overnight, exchanging terms like “machine learning” and “data science” for “AI.”
While OpenAI’s launch incited an LLM arms race among tech titans like Google, Amazon, and Meta, some prominent technologists, like Elon Musk and Steve Wozniak, signed an open letter warning about the ominous future of an unchecked artificial intelligence, urging all AI labs to halt their experiments until our regulatory apparatuses (and our ethics) could catch up. On the pixelated pages of the New York Times and Substack, public intellectuals openly grappled with moral quandaries posed by the specter of an omnipotent artificial intelligence.
Although AI’s most zealous boosters and fearful doomers both likely overstated the capabilities of LLMs and the velocity of research developments in the field, they spurred a set of important ethical questions about the role of technology in society. Posing these questions in the future tense about what we should do if and when tech reaches a distant hypothetical point, though, abdicates our responsibility in the present regarding the ways we are currently using tech and how this reliance might already be imperiling humanity.
We should perhaps be especially vigilant about the use of technology for cybersecurity and warfare, not only because of the obvious moral stakes but also because OpenAI recently appointed a retired US Army general and former National Security Agency (NSA) advisor to its board. The best way to prepare for a perilous future brought about by machines is to observe that that future is already here. And it’s playing out in Gaza.
Israel’s War on Gaza
In a series of groundbreaking investigations, Israeli publications +972 and Local Call have shed light on the extensive role of AI in Israel’s military campaign against Gaza that began on October 8, 2023, which Israel calls Operation Swords of Iron. Relying heavily on testimony provided by six anonymous sources within the Israel Defense Forces (IDF), all of whom have had direct experience with this technology, investigative reporter Yuval Abraham describes three algorithmic systems used by the IDF: “The Gospel,” “Lavender,” and “Where’s Daddy?” Although we cannot independently verify any of the claims made by Abraham’s sources, we will assume their veracity throughout this piece.
According to Abraham’s sources, the Gospel generates a list of physical structures to target, and Lavender generates a list of humans to target. “Where’s Daddy?” is an auxiliary tracking system that is then used to predict when Lavender-generated targets have entered their homes so that they can be bombed.
All of Abraham’s sources, reserve soldiers who were drafted after October 7, have indicated that these systems, when in use, have very little human oversight, with soldiers often providing a rubber stamp of the model output (the IDF has denied these claims). Across two investigations, Abraham suggests that these systems are at least partly responsible for the unprecedented scale of destruction of the current military offensive, especially during the first several weeks.
Indeed, the IDF boasted dropping four thousand tons of bombs on the Gaza Strip in the first five days of the operation. By their own admission, half of these bombs were dropped on so-called power targets. Power targets are nonmilitary civilian structures, such as public buildings or high-rise apartments, located in dense areas that, if bombed, can cause considerable damage to civilian infrastructure. Indeed, they are chosen precisely for this reason.
The logic behind power targets can be traced back to the Dahiya doctrine, a military strategy legitimizing disproportionate civilian destruction during Israel’s war with Hezbollah in 2006 championed by the IDF commander at the time, Gadi Eisenkot. Although the IDF did not officially make use of such power targets against Palestinians until their military campaign against Gaza in 2014, the Gospel system has allowed for the Dahiya Doctrine to be implemented at a more massive scale, generating targets at a faster rate than they can be bombed while maintaining some international credibility against claims of indiscriminate bombing.
The IDF spokesperson Daniel Hagari succinctly reiterated the Dahiya doctrine on October 10, 2023: “We’re focused on what causes maximum damage.” This mirrored Eisenkot’s original summation in 2008: “We will apply disproportionate force . . . and cause great damage and destruction there.” Eisenkot served on the Israeli war cabinet formed by Benjamin Netanyahu on October 11 last year, until he resigned shortly after Benny Gantz did in June 2024, spurring Netanyahu to dismantle the cabinet.
The principle of proportionality aims to prevent the excessive use of force against civilians, one out of proportion with the military gains sought, and is one of the fundamental principles of international humanitarian law. In practice, violations of this principle are difficult to prove, unless they are being proudly broadcasted by the perpetrators.
It is unclear to what extent the IDF is still using the AI technology described in +972 in the current phase of its military operation. It is also unclear how useful such technology would even be in the current phase, given the widespread destruction Israel has already caused, with the majority of homes, hospitals, government buildings, nonprofit offices, and schools damaged or destroyed; electricity largely cut off; and famished, displaced Palestinians frequently moving locations to evade Israeli attacks and find shelter.
However, it is entirely possible that Israel could employ the same systems against Lebanon if a larger conflagration were to erupt. Israel also has a long-running history of selling military technologies to other countries, including other countries that have also committed human rights violations.
The Human-Machine Team
In previous military campaigns in which assassination targets were selected more manually, the selection of each entailed a lengthy incrimination process that involved cross-checking information. While this process was manageable when the pool of targets only included senior-level Hamas officials, it became more cumbersome as the IDF expanded its list of potential targets to include all low-ranking operatives in pursuit of its stated goal to eradicate Hamas. Israel used this goal, however lofty, as a justification for the use of AI to automate and expedite the process of generating targets.
Lavender is a model that is trained to identify any and all members of Hamas and Palestinian Islamic Jihad (PIJ), regardless of rank, with the explicit goal of generating a kill list. The Lavender model that Abraham’s sources described is very similar to what the head of the IDF’s elite Unit 8200 described in both a self-published 2021 e-book entitled The Human-Machine Team: How to Create Synergy Between Human and Artificial Intelligence That Will Revolutionize Our World, under the nom de plume Brigadier General Y. S., and a presentation he gave at an AI conference hosted by Tel Aviv University in early 2023.
Given the highly sensitive nature of Unit 8200’s work, the identity of its commander is usually classified for the duration of their tenure. However, the current commander’s identity, Yossi Sariel, was ironically compromised through the commander’s own poor cybersecurity practices, as his self-published e-book was linked to his personal Google account.
Abraham obtained slides from Sariel’s 2023 AI presentation at Tel Aviv University that include a pictorial representation of a machine-learning model, which was apparently first deployed in Israel’s 2021 military campaign in Gaza. Machine learning is an interdisciplinary field that combines concepts and techniques from mathematics, computer science, and statistics. It builds models that can learn patterns from training data and generalize these findings to new data, often in the form of predictions or other inferences.
Machine learning problems are commonly divided into supervised and unsupervised learning, depending on whether the training data are labeled or unlabeled, or how much supervision the model has when learning from the training data. Sariel’s schematic was presented with the title “PU Learning.” The term likely refers to “positive and unlabeled learning,” a special case of semi-supervised learning problems in which the training data for a classification algorithm only includes labels for the positive class.
The classification algorithm must, therefore, learn how to predict whether a sample is a member of the positive or negative class based on only having access to positive examples in the training data. In this case, the words “positive” and “negative” refer to whether the sample, or individual, is a militant or not. This means that, in the training data (and perhaps in the IDF’s military strategy and ideology more broadly), there is no category for Palestinian civilians.
The features used to classify individuals were collected from a wide array of surveillance sources, likely under the purview of Unit 8200, including phone records, social media, photographs, visual surveillance, and social contacts. The sheer volume of surveillance information that Israel, one of the leading developers and exporters of cybertechnology in the world, collects on Palestinians, especially in Gaza, is astonishing, and makes it extremely difficult to understand why known civilians would not be labeled negative in the training data.
In 2014, forty-three veterans that served in Unit 8200 signed a letter criticizing the unit and refusing further service, saying that, “Information that is collected and stored [by Unit 8200] harms innocent people.” Much of the Israeli government’s data, data storage, and computing power comes from Google and Amazon, afforded by multiyear, multibillion-dollar contracts.
Decision Points
Although models seem precise, in practice they are vague and flexible enough to easily accommodate (and hide) a multitude of intentions. Building an AI system entails an almost limitless set of decision points.
First and foremost, those decision points involve the specification of the problem, the collection and manipulation of training data (including the labeling of samples), the selection and parameterization of the algorithm, the selection and engineering of features to be included in the model, and, finally, the model training and validation process. Each of these decision points is associated with biases and assumptions, which must be thoughtfully considered and balanced alongside each other.
One common assumption for algorithms used for PU Learning problems is that the positive-labeled samples in the training set are selected completely at random, independent of the features or attributes of the samples. Although this assumption is often violated in the real world — as is almost certainly the case for labeling Hamas and PIJ militants — it allows for a greater array of choices among algorithms.
Under this assumption, however, it is not possible to estimate the precision of the model, only the recall. Both statistical measures of accuracy, the precision of a model refers to the proportion of positive predictions that are, indeed, according to the ground truth, truly positive, taking into account any false positives the model made, whereas the recall of a model refers to the proportion of positive samples in the data that the model correctly classifies.
A hypothetical model that predicted all samples to be positive would have perfect recall (it would correctly identify the entirety of the positive class) but poor precision (it would incorrectly classify the entire negative class a positive, generating many false positives). Given that the stated objective of Israel’s government since October 7 has been to eradicate Hamas, one can only assume that the IDF — even though it has recently distanced itself from this objective — is more concerned with optimizing the recall of the model rather than its precision, even if this comes at the expense of generating more false positives (failures to detect civilians as such).
Indeed, multiple statements by Netanyahu and former Israeli war cabinet members — not to mention the collective punishment and wholesale destruction of conditions of life in Gaza — cast doubt on whether innocent Palestinians even exist as an ontological category for the IDF. If there are no innocent Palestinians, any measurement of model precision is superfluous.
Concerningly, Abraham’s sources imply that the training set includes not only known Hamas and PIJ militants but also civil servants working in Gaza’s security ministry who help administer the Hamas-run government but are not Hamas members, nor indeed political activists at all. It would not be surprising for the IDF to label civil servants working in a Hamas government as enemies of Israel, given the IDF’s notoriously broad definition of terrorist. But this would also create a serious problem for the model, assuming it was in fact only trained on positive and unlabeled data. If these samples were labeled as positive, it would mean that the model would learn to associate features shared among civil servants with the positive class, resulting in misclassifications.
Indeed, according to Abraham’s sources, Lavender erroneously targets civilians who share the same name as a suspected militant, have communication patterns that are highly correlated with suspected Hamas militants (like civil servants), or have received a mobile phone that was once associated with a suspected Hamas militant. And although the model is known to make errors approximately 10 percent of the time, the output is not scrutinized beyond the occasional need for confirming that the targets are male (in general, most males, even children, are assumed to be militants by the IDF).
Because the model predicts the probability that a given individual is a militant, the list of targets, or presumed militants, can be shortened or elongated by changing the threshold at which this probability is converted into a binary variable, militant or not. Is someone with a 70 percent likelihood assumed to be a militant? Just over 50 percent? We don’t know, but the answer may change from day to day or week to week.
In practice, you can adjust the threshold to generate the number of targets you desire, whether because, as one of Abraham’s sources explained, you are being pressured to “bring . . . more targets” or because you need to temporarily scale back due to US pressure.
Kill Lists
Although we do not know the range of thresholds used, or the range of probabilities generated by the model, we do know, thanks to Abraham’s reporting, that the thresholds were indeed regularly altered, sometimes arbitrarily and sometimes explicitly to make the list longer. These changes were often made by low-ranking military officers who have very little insight into the model’s inner workings and are not authorized to set military strategy.
This means that low-ranking technicians are empowered to set the thresholds at which people live or die. Given that targets are intentionally bombed in their homes, changing a particular threshold by a small margin could plausibly lead to a manifold increase in civilian casualties.
By changing the features, the training data, or — most simply and most easily — the probability threshold at which people are classified as militants, one could configure the model to deliver essentially whatever output desired while maintaining plausible deniability that it was based on a biased, subjective decision.
The kill list generated by Lavender is fed into an auxiliary system, Where’s Daddy?, that tracks these human targets across the Gaza Strip and detects the moment when they enter their respective family residences. Although in the past the IDF has only bombed senior military officials in their homes, all targets — including presumed low-level Hamas operatives and, inevitably, due to model error, mislabeled Palestinian civilians — have been intentionally bombed in their homes during Operation Swords of Iron.
Intentionally bombing homes where there is no military activity directly violates the principle of proportionality. Doing so inevitably leads to an increased civilian death toll and conflicts with the IDF’s persistent claim that Hamas’s use of civilians as “human shields” is to blame for the death toll in Gaza.
Abraham also makes the point that these interlocking systems — and the human decisions that undergird them — could be why dozens of families have been entirely wiped out. Whether it was the explicit intention of IDF officials to annihilate entire families seems of little relevance if there is a procedure in place that systematically does so.
Moreover, the decision was made to use unguided “dumb” bombs when bombing these targets, resulting in even more collateral damage, or civilian casualties, despite the fact that Israel has one of the most advanced and well-supplied militaries in the world. The IDF justified this decision on the basis of conserving expensive armaments like precision-guided missiles, though it does so directly at the cost of sacrificing more human lives. Israel cares more about saving money on bombs (of which it has an almost infinite supply) than saving the lives of innocent Palestinians.
Technological Cover
It’s clear that Operation Swords of Iron does not rely on these systems for precision — the civilian death toll plainly indicates that neither the AI-generated targets nor the weaponry is precise (of course, this means taking Israel’s goal of eradicating only militants at face value). What they provide, rather than precision, is scale and efficiency — it’s much easier and faster for a machine to generate targets than a human — as well as providing technological cover to hide behind.
Because no one person is responsible for the target generation, everyone involved in the operation has plausible deniability. And because it can be easier to place trust and faith in a machine, to which we often misattribute infallibility and objectivity, than an imperfect human rattled with emotions (indeed, this is corroborated by some of Abraham’s sources), we might also place less scrutiny on a machine’s decisions.
This is the case even if those machine decisions can more accurately be viewed as human decisions implemented and automated by a machine. Indeed, although the soldiers interviewed by Abraham suggest these systems have replaced human decision-making, what these systems actually seem to have done is obscure it.
It was a human decision to allow “collateral damage” of up to twenty people for presumed junior-level targets and up to a hundred people for presumed senior-level targets. It was a human decision to bomb the homes of junior-level targets, to optimize for recall and not precision, to deploy a model with at least a 10 percent error rate, and to implement arbitrary thresholds that determine life and death.
Human beings decided to use highly confounded data to generate features for the model, to label civil servants as Hamas militants, and to “eliminate the human bottleneck” in target generation so as to generate targets at a faster rate than they could be bombed. They also decided to target civilian structures in dense areas and use unguided bombs to strike these targets, creating even more collateral damage.
Dehumanization and diffusion of responsibility play a powerful role in explaining how atrocities can be committed, especially at scale. By reducing an entire population to a vector of numbers and using machines to build a “mass assassination factory,” these AI systems help provide some of the psychological mechanisms necessary to commit the kinds of atrocities we have seen play out over the last nine months in Gaza.
Outsourcing Control
The IDF hides its genocidal logic behind a sophisticated veneer of technology, hoping that this will lend some legitimacy to its military actions while obscuring the brutal reality of its asymmetric warfare. It did exactly this in mid-October 2023, when Israeli officials gave New York Times reporters limited access to output from their data-tracking systems, “hoping to show that it was doing what it could to reduce harm to civilians.” In the first month of the war, over 10,000 Palestinians were killed by Israel, including 4,000 children and 2,700 women.
We should take seriously the discrepancy between the “human-machine team” that Brigadier General Y. S. envisions in his book and the AI systems deployed under Yossi Sariel’s leadership. What an idealized model does in theory, which often seems reasonable and benign, will always be different from what the model does in practice.
What separates them, in large part, is a cascade of obscure human decisions and external exigencies, some seemingly trivial, some implicit, some significant. Models are not morally responsible for the automated decisions they make; the human agents who select, train, and deploy them are, as are the humans who idly let it happen.
As AI becomes more integral to the way our everyday lives and our broader societies function, we should ensure that we aren’t ignoring or abdicating moral responsibility for what AI models are doing. And as generative models like ChatGPT open the door to the automation of machine learning itself, we should be especially wary.
The most urgent and pressing danger of AI is not that machines will develop runaway intelligence and begin to act outside of human control. It is that we, as humans, will become too dependent on AI, voluntarily outsourcing our own decision-making and control. As ever, we are the biggest threat to our humanity, not machines.